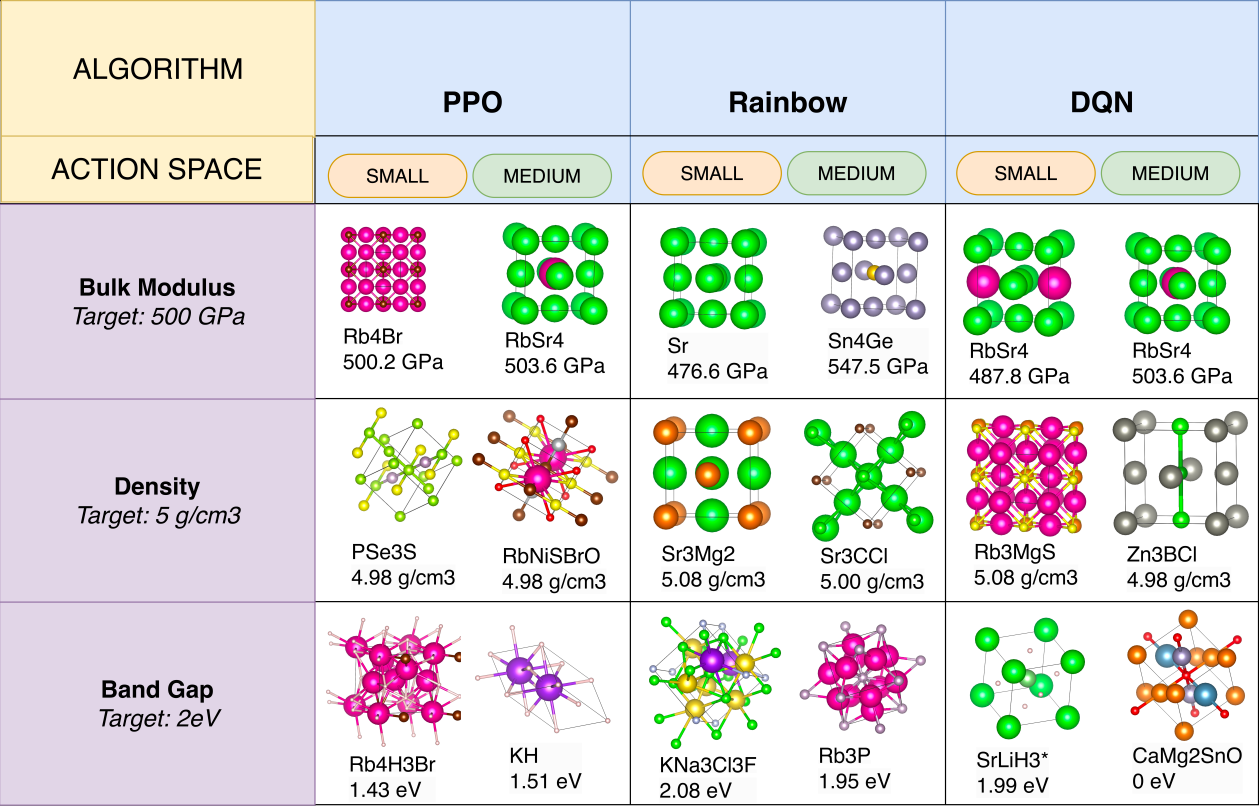
CrystalGym: A New Benchmark for Materials Discovery Using Reinforcement Learning
CrystalGym pioneers RL for material design by embedding expensive DFT calculations for property predictions directly into training—avoiding approximations used in generative methods. While current algorithms struggle with all tasks (e.g., band gap optimization), the environment establishes a benchmark for RL in noisy, slow-reward domains, focusing on problem aspects crucial for molecular generation.
Prashant Govindarajan, Mathieu Reymond, Antoine Clavaud, Mariano Phielipp, Santiago Miret, Sarath Chandar
AI for Accelerated Materials Design Workshop @ ICLR 2025 (to appear)
AI for Accelerated Materials Design Workshop @ ICLR 2025 (to appear)